The Computer Vision and Machine Perception Lab at the University of Technology in Nuremberg focuses on 3D computer vision and lifelong learning. The emphasis lies on building machine perception algorithms that are not rigid but able to adapt to their environment and evolve. The lab connects to surrounding interdisciplinary fields, such as fundamentals, reinforcement learning, natural language processing, and privacy. The lab is headed by Prof. Dr. Eddy Ilg, who is known for his contributions to computer vision on optical flow and his work on AR technology at Meta.
Team Computer Vision and Machine Perception Lab
News
1.10.2024 | The CVMP Lab is moving to Nuremberg. |
23.8.2024 | Eddy Ilg was awarded a full professorship at UTN. |
11.7.2024 | Two of our papers were accepted to ECCV 2024: latentSplat and iNeMo: Incremental Neural Mesh Models for Robust Class-Incremental Learning. |
6.5.2024 | Eddy Ilg became an area chair for NeurIPS. |
3.5.2024 | Our paper Neuroexplicit Diffusion Models for Inpainting of Optical Flow Fields was accepted to ICML. |
25.3.2024 | Our State-of-the-Art Report on Recent Trends in 3D Reconstruction of General Non-Rigid Scenes was accepted to the Eurographics (STAR) and Computer Graphics Forum 2024. |
20.3.2024 | Four of our papers were accepted to CVPR 2024: Neural Point Cloud Diffusion for Disentangled 3D Shape and Appearance Generation, Neural Parametric Gaussians for Non-Rigid Reconstruction, Unsupervised Learning of Category-Level 3D Pose from Object-Centric Videos, and Accurate Training Data for Occupancy Map Prediction in Automated Driving using Evidence Theory. |
Publications
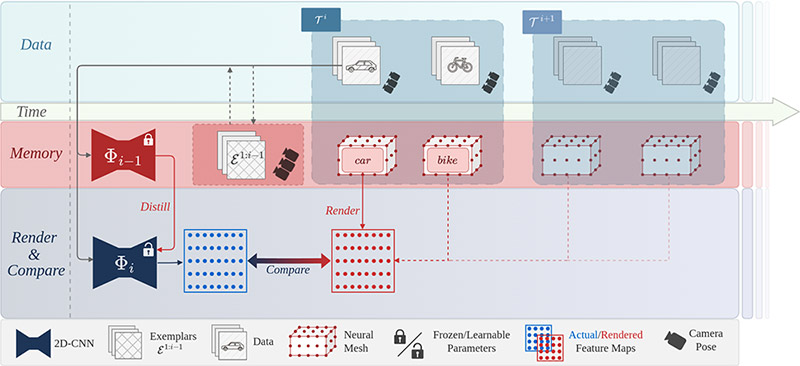
iNeMo: Incremental Neural Mesh Models for Robust Class-Incremental Learning (ECCV 2024)
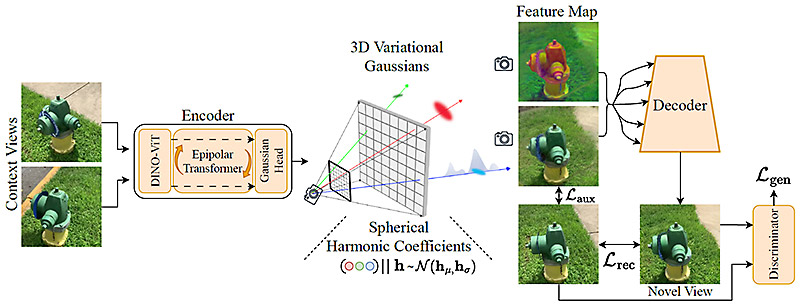
latentSplat: Autoencoding Variational Gaussians for Fast Generalizable 3D Reconstruction (ECCV 2024)
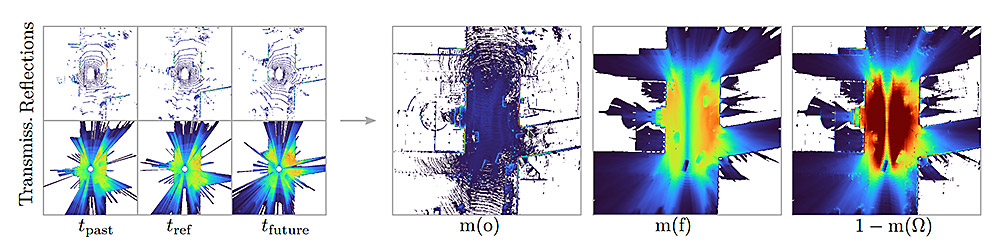
Accurate Training Data for Occupancy Map Prediction in Automated Driving Using Evidence Theory (CVPR 2024)
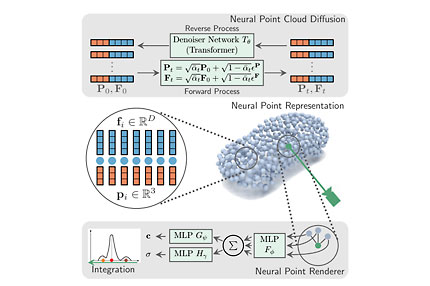
Neural Point Cloud Diffusion for Disentangled 3D Shape and Appearance Generation (CVPR 2024)
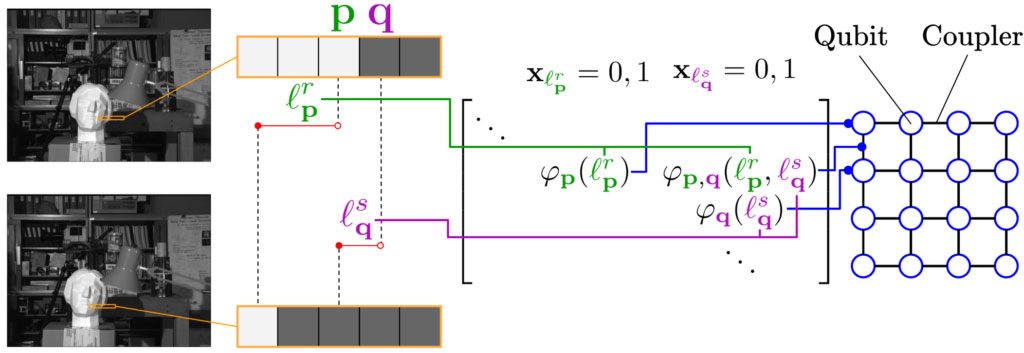
Quantum-Hybrid Stereo Matching With Nonlinear Regularization and Spatial Pyramids (3DV 2024)
List of publications of Prof. Dr. Eddy Ilg on Google Scholar